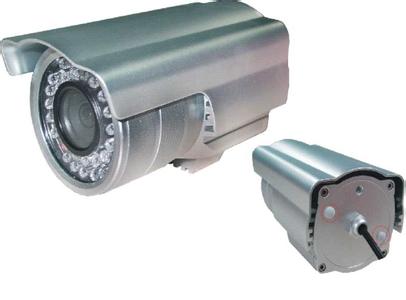
There are two aspects of video surveillance data - massive and unstructured. The amount of video surveillance data is huge, and with the trend of high-definition and ultra-high-definition, the video surveillance data scale will grow at a faster exponential level. Unlike the commonly-used structured data, the video surveillance service generates vast amounts of data. Most of them are based on unstructured data, which brings great challenges to traditional data management and usage mechanisms.
The predicament of data flooding for video surveillance is rapidly increasing video surveillance data, which makes traditional video surveillance system architecture, data management methods, and data analysis applications face new challenges.
Dilemma one, the contradiction between the sharp increase in data volume and IT investment. According to the rules of the IT industry: Under the premise of meeting customer needs, the lower the technology cost, the stronger its vitality. Due to the rapid expansion of data volume and the increasing demand for large-scale computing, the use of high-grade hardware has made hardware investment unaffordable for customers. Customers increasingly expect to meet the demand. Replace high-level hardware with low-end and mid-range hardware.
Dilemma 2: Contradictions between massive data and effective data. The camera works 24 hours a day, 24 hours a day. Truthfully recording everything that happens in the lens coverage. It is not enough to just record the information because most of the information may be invalid to the customer. The valid information may only be distributed in a short period of time. In statistical terms, information is presented as a power-law distribution, also known as the density of information, and often the higher the density of information, the greater the value to the customer.
Dilemma 3, the contradiction between resource utilization and efficiency, the contradiction between serial computing and parallel computing. After the video surveillance service is networked and networked, there are more and more devices in the network. Utilizing idle computing resources to maximize resource utilization is related to the efficiency of operations. In the field of video surveillance, the efficiency of video analysis often determines the value. Lower delays and more accurate analysis are often the general needs of such customers as Pingan City. With the increase of data volume, even if data of TB-level data is analyzed and retrieved for video content, the serial computing model may take several hours to calculate, and it is far from being able to meet the requirements of timeliness. The analysis and retrieval of videos cannot rely on traditional means. The efficiency of huge amounts of data is optimized. Parallel computing is the only way out of video intelligence analysis.
Big Data Key Technology Profile Because big data has brought a lot of practical problems, in order to solve these problems we need new technological changes. We need a new generation of database technology, and the industry calls it big data technology. IDC is defining big data technology: big data technology will be designed to be used for very rapid (velocity) acquisition, discovery, and analysis, from volumes, to multiple categories (variety) The value of the data extracted will be a change in the technology and architecture of the next generation of IT. It is in this context that Hadoop technology was born. After several years of accumulation, Hadoop has grown into a powerful ecosystem. Not only has HDFS, HBase, Hive, and other subprojects been derived, it has become a widely used big data model framework in IT.
The integration of big data technology and video surveillance services in the architecture of the system "In addition to God, anyone must use the data to speak," the famous American management scientist and statistician Edward Deming raised the data to a level parallel to God. Video surveillance business is a typical data-dependent business, relying on data to speak. It can be said that big data and video surveillance services have a natural combination. In a comprehensive view, the combination of big data and data monitoring services is mainly reflected in "storing," "seeing," and "using."
"Flash memory": If the analogy of reservoir water storage, typical network video surveillance data storage model is a water storage method that gathers rivers from small streams and then converges to reservoirs. The increase of the number of streams and the increase of water volume are the guarantees of reservoir water storage capacity. However, the increase of water storage capacity in the traditional way will increase the requirements for reservoir construction costs and water storage safety. The use of a distributed water storage model to establish a number of intermediate reservoirs in the middle reaches of rivers not only reduces the pressure and cost of water storage in the main reservoir, but also improves the efficiency of water use in the vicinity. Under the support of big data technology, the network video surveillance data storage model can be turned to a distributed data storage system to provide efficient, secure, and inexpensive storage.
"Easy to see": In the video surveillance business, misunderstandings, misses, and late watching are common problems. The backtracking of big data monitoring images has brought many physiological and psychological challenges to many security monitoring and management personnel. In the retrospection of a large number of manpower-intensive public security cases, it is often heard that “seeing vomiting†and “seeing dizzy†are frustrating and sighing. It can be imagined that the general retail industry, financial industry, etc., are more difficult to trace back to video surveillance images. With the advent of big data trends in video surveillance, relying on the human eye to retrieve and view all video image data is not realistic. The use of big data technology to achieve fuzzy video image query, fast retrieval, accurate positioning, let the look becomes simple and imminent.
"Useful use": In the video surveillance business, it is only one of the information collection methods. The use is the fundamental of business applications. The efficiency of video surveillance services has become a key bottleneck that hinders the development of the industry. As the coverage and coverage of video surveillance cameras increase, the amount of video data increases exponentially, while the efficiency of video surveillance data usage decreases. The emergence of integrated video surveillance and image intelligence analysis services such as intelligent transportation applications and consumer behavior analysis applications is trying to break through the bottlenecks of video surveillance efficiency value and low commercial value. Through the big data technology, the value information behind the massive video surveillance data is further excavated, and the rapid feedback of connotation knowledge to aid decision-making is the golden key for using video surveillance well and using goodness.
Video surveillance architecture for big data The core of the video surveillance business is data, and the data is the business itself. Based on the big data architecture, it can bring many benefits to medium and large-scale video surveillance projects.
First, the architecture is more flexible and scalable. For some medium and large-scale projects, due to the difference in starting points and the lack of top-level design of the video surveillance architecture, the later expansion and upgrading will inevitably end up. For example, the big data-oriented architecture will be introduced in the early stage of construction, which will bring benefits to business expansion and management. Second, to meet the explosive growth of video surveillance data with cheaper general-purpose hardware. In the big data-oriented architecture, multiple HDFS clusters can be set up to meet the deployment needs of video surveillance services. The collected flow data is divided into segments and distributed over data nodes. These data nodes can use inexpensive general-purpose hardware. High-reliability is ensured by software technology. This approach avoids the use of traditional high-end hardware models and greatly reduces investment costs. Third, intelligent analysis and data mining are achieved through high-speed parallel computing. For the gold mines, only the bright gold is valuable. The video surveillance data is just like such a gold mine. Traditional manual and serial data screening methods have not met the requirements in the era of big data. The architecture principle for big data is to decompose massive data into smaller, more accessible, batch data and perform parallel analysis and processing on multiple servers, thereby greatly accelerating the processing of video data.
Combining the features of video surveillance services, introducing the architecture of Hadoop and building a big data video surveillance architecture from the perspective of top-level design will have a profound impact on the planning and design of future video surveillance services. The following is a concise description of the following logical architecture for monitoring big data video.
The data source layer includes real-time data and non-real-time data.
Real-time data refers to real-time streaming media data generated by IP cameras and sensors. Non-real-time data refers to media data imported from DVRs, encoders, and third-party systems.
The big data storage layer adopts HDFS and HBASE to realize low-cost, high-reliability management of data. Store the collected streaming video in the HDFS cluster and set up an access index through HBase. Reconstruct the traditional NVR and dedicated storage into the overall distributed file system.
Big data computing layer, intelligent analysis and data mining. Decompose the analysis of large video by MapReduce, make full use of idle resources, and transfer computing tasks to multiple servers for parallel computing analysis. On the other hand, according to the video metadata generated by intelligent analysis, the value of video metadata can be mined through Hive. information.
Business and management, equipment and business management. The server cluster based on Zookeeper can ensure the trouble-free operation of the business system and implement the supervision of cameras and other devices based on Ganglia.
The video architecture based on big data is essentially a technical architecture that uses video data as the most valuable asset and uses data as the core. It focuses on solving the problem of massive video data decentralization, centralized storage coexistence, and multi-level distribution. This greatly improves the efficiency of reading and writing unstructured video data and provides an end-to-end solution for fast retrieval and intelligent analysis of video surveillance.
The Value of Big Data Video Surveillance Architecture The Big Data Video Architecture is a revolutionary technology, especially in the field of real-time intelligent analysis and data mining, allowing video surveillance from manual sampling, progress to high-efficiency pre-warning, post-mortem analysis, and intelligent information Analysis and prediction bring profound changes to the business of video surveillance:
Safe urban areas, real-time summary and comprehensive analysis of various public safety data and data to provide scientific basis for law enforcement officers to quickly and accurately respond: such as real-time access to live video footage, criminal suspect records, similar case information in the same region; A comparative analysis of time and space reveals its criminal patterns and behavioral patterns; it tracks the location of suspects and their vehicles. Commanders can also conduct comprehensive analysis of data from different sources with reference to various data to create a command plan.
The intelligent transportation industry can easily monitor the driving status and running trajectory of all vehicles within the camera coverage, and quickly analyze whether it is illegal or not. Through the comparison, analysis, and judgment of mass traffic data, the vehicle's driving path and road congestion can be determined. Other functions.
Cloud services: Realize the video surveillance cloud service based on big data, let the cameras connect to cloud video surveillance hosting services through the Internet, and quickly and intelligently analyze big data deployed in the cloud for small businesses, retail stores, and restaurants. Hotels and other real-time monitoring video and potential risk management can even provide a fee-based video content-based analysis reports, such as the number of daily customers, the average queue length, etc., to create new business models.
We specialize in supplying quality drilling equipments PDC Drag Bit , double cutter pdc Drag Bit , single row cutters pdc drag bit ,3-wings drag bits, 4-wings drag bits, 5-wings drag bits, claw type, clay bits, for mining, water-well drilling, seismic prospecting for many years.
PDC drag Bit Description: Silver solder welded composite sheet, does not fall off and does not collapse. The outer wing of the blade is covered with a half-grain composite sheet to protect the blade from small wear and wear, and the large outlet hole does not contain swarf and quickly discharges cuttings. Multi-blade balance strength, smooth and fast drilling, national standard precision thread buckle, quick and easy loading and unloading, not falling off.
Thread standard: national standardProduct categories: 65mm, 75mm, 91mm, 113mm, 133mm, 153mm, 171mm, 219mm above are the general standards, if you have special needs 311mm,346mm , 490mm, 500mm etc, you can order.
PDC drag bit advantage
1.Small or medium PDC cutter layout.
2. Multiple blade profile dasign,available with round,double laper and parabolic profiles.
3. Various ID flow path desighs provide suffcient cooling and cleaning to whole bit surface.
4. Applicable stratum: soft rock layers such as sandstone, mudstone and weathered rock, with fast footage and long service life.
3 blades PDC drag bit box thread
Double row cutters 5 blades PDC drag bit
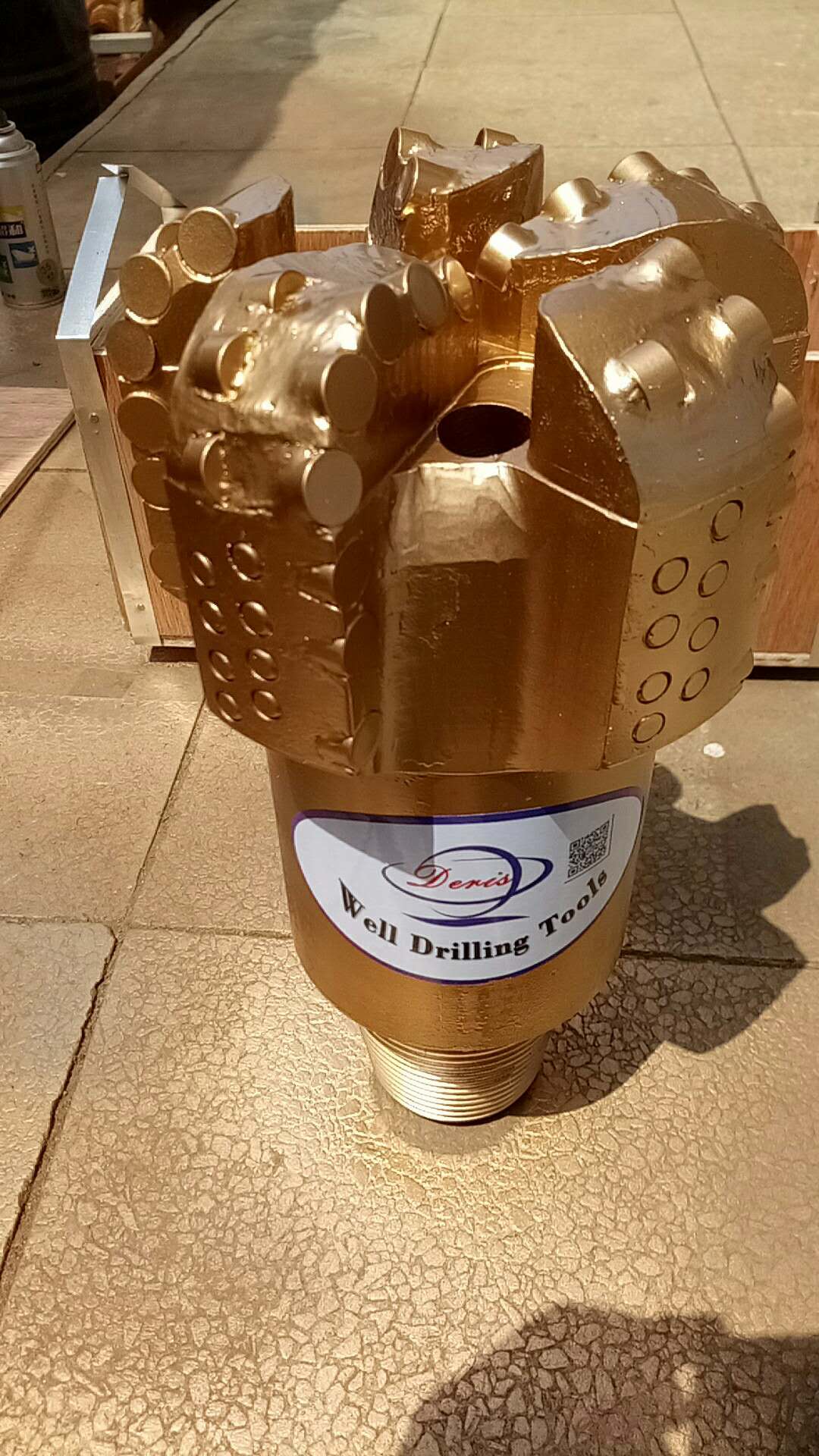
6 blades PDC Bit
PDC Drag Bit
PDC Drag Bit,PDC Drag Drill Bits,Three Wings PDC Drag Bit,4 Blades PDC Drag Bit
Hejian Deris Petroleum Drilling Equipment Co.,Ltd. , http://www.welldrillingbit.com